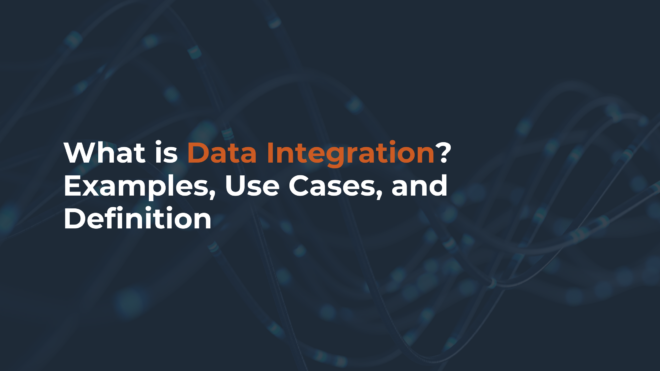
Demystifying Data Integration: What Do We Mean By It?
Data integration is the process of combining data from multiple sources into a unified view. It’s like taking all the puzzle pieces scattered on the floor and assembling them into a complete picture. Using a data integration tool can help you make more informed decisions and streamline operations in ways that wouldn’t be possible with isolated data sets.
Think of it like this: imagine your sales team has customer data in their CRM, the marketing team has email campaign data in their platform, and finance has transaction data in another system. Integrating these siloed data sources allows you to understand the complete customer journey, identify buying patterns, and optimize marketing campaigns based on actual sales performance. That’s the magic of data integration!
Beyond Buzzwords: Data Integration in Action
Now, let’s get practical. Let’s explore some real-world examples of data integration:
- Customer 360: Combining data from multiple sources like websites, social media, and loyalty programs to create a single, unified view of each customer, enabling personalized marketing and improved customer experiences.
- Real-time fraud detection: Integrating transaction data from multiple systems (payment gateways, bank accounts, etc.) in real-time to identify and prevent fraudulent activity instantly.
- Inventory management: Synchronizing inventory data across warehouses, stores, and online platforms to ensure optimal stock levels and prevent product shortages.
- Operational efficiency: Integrating data from production lines, maintenance systems, and quality control processes to optimize manufacturing processes and reduce downtime.
These are just a few examples, and the possibilities are truly endless.
What is data integration vs ETL?
While often used interchangeably, ETL (Extract, Transform, Load) is actually a specific data integration technique. ETL focuses on extracting data from source systems, transforming it into a consistent format, and then loading it into a target data store (like a data warehouse). Think of it as cleaning and organizing the puzzle pieces before fitting them together.
Data integration encompasses a broader range of approaches, such as data replication, application integration, ETL, real-time data integration, data virtualization, and more. So, while ETL is a tool in the data integration toolbox, it’s not the only tool available.
Four Approaches to Data Integration
Now, let’s dive into the four main types of data integration methodologies:
- ETL (Extract, Transform, Load): We’ve already covered this workhorse, ideal for batch processing and data warehousing.
- ELT (Extract, Load, Transform) is one type of data management process. A modern twist on ETL, ELT loads data into a flexible repository (like a data lake) before transformation, allowing for greater flexibility and handling of unstructured data.
- Data Virtualization: This approach creates a virtual layer on top of existing data sources, providing a unified view without physically moving the data. Think of it as a real-time map that guides you through different data silos.
- Real-time Data Integration: As the name suggests, this focuses on integrating data streams in real-time, enabling immediate reactions to events and insights. Think of it as watching the puzzle being assembled piece by piece, gaining insights as the picture unfolds.
Choosing the right data integration tool depends on factors like your data needs and business processes. budget, and technology infrastructure. But one thing’s for sure: there’s a data integration method out there for everyone!
Beyond the Basics: Deep Dives into the Data Integration World
This blog post is just the tip of the iceberg. Here are some key areas we can explore further:
- Integration Tools and Platforms: Delving into the powerful tools and platforms that automate and simplify data integration tasks, making it accessible even for non-technical users.
- Big Data Integration: Tackling the challenges of integrating vast amounts of diverse data, including unstructured and semi-structured formats.
- Change Data Capture: Understanding how this technology captures data changes in real-time, enabling near-instantaneous insights and reactions.
- Data Governance: Exploring the crucial role of data governance in ensuring data quality, compliance, and security throughout the integration process.
- Benefits and Challenges: Weighing the advantages of data integration (improved decision-making, operational efficiency, etc.) against potential challenges like data quality issues and integration complexity.
Like any powerful tool, data integration boasts immense benefits while presenting unavoidable challenges. Weighing these two sides of the coin is crucial for making informed decisions about your data strategy.
Benefits:
- Enhanced decision-making: By having a unified view of data, you can draw deeper insights, identify trends, and make data-driven decisions that are more likely to succeed. Imagine having all the puzzle pieces – you can finally see the complete picture and make informed choices about your next move.
- Improved operational efficiency: Data integration streamlines processes, eliminates data silos, and automates tasks, leading to increased operational efficiency and cost savings. Think of it like removing roadblocks and creating a smooth highway for your data to flow, resulting in faster and more efficient operations.
- Boosted customer satisfaction: By leveraging integrated data, you can personalize customer experiences, anticipate needs, and resolve issues faster, leading to happier and more loyal customers. Imagine having a complete understanding of each customer – you can tailor your interactions and services to truly delight them.
- Competitive advantage: Data-driven insights gained through integration can help you identify market trends, develop innovative products, and optimize your offerings, giving you a leg up in the competitive landscape. Think of it as having a secret weapon – the ability to analyze and react to market changes faster than your competitors.
Challenges:
- Data quality issues: Integrating data from different sources can often mean dealing with inconsistencies, inaccuracies, and missing values. Ensuring data quality throughout the process requires careful cleaning and transformation efforts. Think of it like sifting through sand to find the gold – you need to remove impurities and refine the data before it becomes truly valuable.
- Integration complexity: The sheer volume and diversity of data sources can make integration a complex undertaking, requiring specialized skills and powerful tools. It’s like building an intricate bridge – you need the right tools and expertise to ensure it’s stable and efficient.
- Security and privacy concerns: Combining data from disparate sources raises concerns about data security and privacy. Implementing robust security measures and adhering to data regulations are crucial to ensure responsible data integration. Think of it as building a secure tunnel for your data to travel through – you need to protect it from unauthorized access and ensure privacy compliance.
- Cost and resource investment: Implementing and maintaining data integration solutions requires financial and resource investment. It’s like building a new road – you need to invest in construction and maintenance to ensure it remains functional and valuable.
By carefully considering both the benefits and challenges, you can determine if data integration is the right strategy for your organization. Weighing the potential gains against the investment and implementation hurdles will help you make a well-informed decision that aligns with your data goals and resources.